|
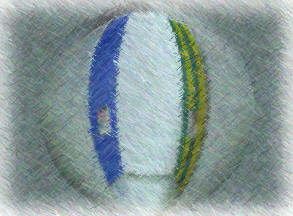
Geometry, Features, and Orientation in Vertebrate Animals: A Pictorial
Review
Abstract
With much pictorial support, we review research on how
vertebrate animals orient in enclosed rectilinear spaces.
The phenomenon of the rotational error is introduced, along
with the distinction between geometric and featural information.
The circumstances under which a number of species use geometric and featural information for relocating a target place are reviewed.
Other sections examine cue competition (actually lack thereof) between geometric information and landmark cues, artificial intelligence, and the
neurophysiological bases underlying the coding of geometric information. We then examine two theoretical issues: 1) how geometry is encoded and used,
2) the modularity of mind. |
Chapter Outline &
Navigation
|
We recently reviewed this literature in print (Cheng & Newcombe, 2005). Here we present an updated and far more pictorial review.
To take full advantage of the web format, results and theory are discussed briefly in words, in
'nugget form'. Each section is
illustrated by appropriate pictures and other visual presentations. The basic phenomenon (Rat section) and the distinction between geometric and featural information are crucial for understanding the entire literature. We suggest that you read this section first. Otherwise, the following
sections on data can be read in any order as you wish. We suggest, however, that you read at least a few of them before proceeding to the
theoretical sections.
The idea of the use of the geometry of surfaces started with
the doctoral work of Ken Cheng. Below is a picture of the
rectangular arena used to test the animals, male white rats (see
Figure 1). The photo was taken outside, not in lab conditions. The
arena measured 120 cm by 60 cm. Not easy to notice in the photos are
two additional kinds of cues: different textures on the corner
panels and smells emanating from two of the corners. In the
experiments, the bottom of the arena was filled with saw dust (wood
chips used for rat bedding). A grid was drawn on the bottom for the
purposes of scoring where the rats dug.
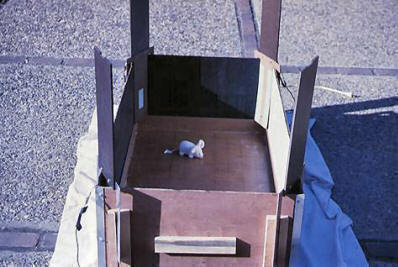 |
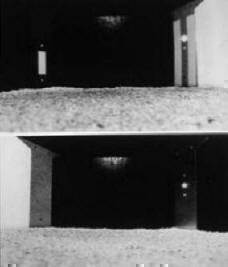 |
Figure 1.
A view of the rectangular arena used to test male white
rats..
|
Figure 2.
These photos, of the two ends of the rectangular arena,
were taken in the lab, from the floor of the box.
|
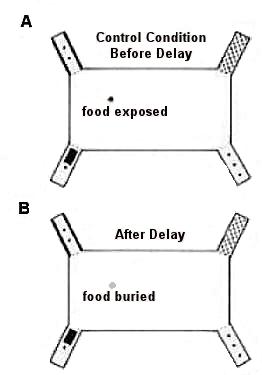 |
Figure 3. Schematic illustration of the basic task.
|
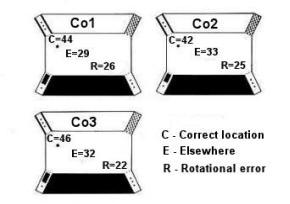 |
Figure 6. Results from Cheng (1986).
|
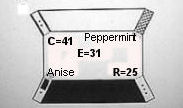 |
Figure 7. Cheng (1986) results averaged over the
three rats.
|
The task was a
working memory find-the-treasure-again task. Figure 2
represents roughly what a rat saw in the tasks. A box (12 X 6
cm) was put on the bottom of the arena at a randomly chosen
location (Figure 3). In the exposure phase, the box
was exposed, revealing 7 pellets of sweet cereal. The rat got to
eat 3 pellets, and then was removed outside the room. The arena
(actually an identical looking replica) was rotated at
random, the remaining food was buried under saw dust, and
the rat was returned to the arena 90 s later to dig up the
remaining food.
The rotational error is
crucial to understanding this entire corpus of work (Figure
4). In Figure 5, A. shows a
plan view of the task situation, with the location of the
food indicated by the filled dot. The open dot shows the
rotational error, located at 180 degrees rotation through
the center from the correct location. B. A record or
representation of the geometric properties, with the food
location indicated by the dot. This representation contains
only the broad shape of the space, called the geometric
properties. Missing are smells, textures, colors of walls,
the information shown in C. Using a record such as B. can
lead to rotational errors. This happens when the top wall of
B. is matched to the bottom wall of of A., with the box in
B. rotated 180 degrees. Using a record of the nongeometric
information, called featural information, shown in C.
results in an unambiguous match. Systematic rotational
errors thus indicate a record such as that shown in B. We
will argue later that far less than the entire geometric
shape is represented by rats.
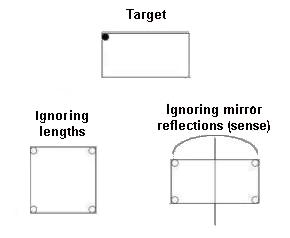 |
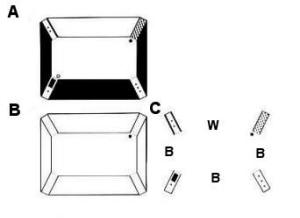 |
Figure 4. Rotational error.
|
Figure 5. Geometry and rotational error. |
Figures 6 and 7 show results of individual rats in the working memory task in
Cheng (1986). C stands for correct choices, R stands for
rotational errors, and E stands for everything else (other
nonsystematic wrong choices or no digging at all). Each rat made
a significant number of rotational errors. The advantage of
correct choices over rotational errors (not statistically
significant in any rat) is attributed to the fact that the rats
were not disoriented during the delay. Margules and Gallistel (1988) repeated this experiment with
disorientation during the delay period. The rats then make
approximately equal numbers of correct choices and
rotational errors. In a
reference memory task (Cheng, 1986, Experiment 2), the food
stayed in one of the corners on every trial (Figure 8). The rats then
learned to choose the correct corner over the rotational
error (diagonal opposite). The rats as a group, however,
still made systematic rotational errors (see Figure 9). This pattern
(correct choice over rotational error, but systematic
rotational errors still) is also found in young children and
rhesus monkeys.
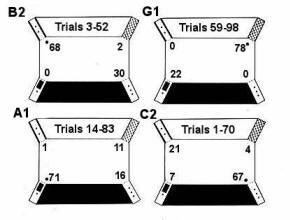 |
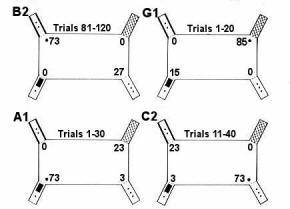 |
Figure 8. Results from reference memory task.
|
Figure 9. Cheng's rats chose the correct corner
over the rotational error even when the white wall
was made black, making the colors of walls
nondiagnostic for locating the food. Again, they
still made systematic rotational errors.
|
Benhamou and Poucet (1998) introduced two differences in the reference
memory paradigm. 1) Their task was aversive rather than
appetitive. Others have noted differences in performance
between appetitive and aversive tasks. Golob and Taube
(2002) found that in a rectangular swimming pool, rats did
not make systematic rotational errors. In a dry, appetitive task in the same arena,
rotational errors were prevalent. 2) The geometry was
defined by an array of objects, not by surfaces. The pool
was round and surrounded by a ring of curtains, rendering
its geometry useless for the task.
In Figure 10, the blue dot at the bottoms of the panels shows the target
position. The landmarks were different from one another
perceptually. In the left panel, the configuration of
landmarks specified the target unambiguously, and the rats
learned to searched preferentially around the target. In the
right panel, the configuration of landmarks left a threefold
ambiguity as to target position. Individual perceptual
identities of the landmarks needed to be used to resolve the
ambiguity. The rats did not learn to resolve this ambiguity
in 75 trials.
This task (Figures 10 and 11) is much
harder than a standard pool task with lots
of room cues, which supply both geometric and featural cues
(Poucet, Lenck-Santini, & Save, 2003). This manipulation
shows that it is the configuration of landmarks, rather than the
individual identities of landmarks, that was important for the
rats. The blue dot at the bottoms of the panels again shows the
target (see Figure 11). When the landmarks swapped position
(right panel), the configuration
of landmarks and the individual identity of the nearest
landmark dictated different responses. Rats mostly 'went
with' the configuration, although their performance was
slightly (and significantly) worse than under standard
testing conditions (Benhamou & Poucet, 1998).
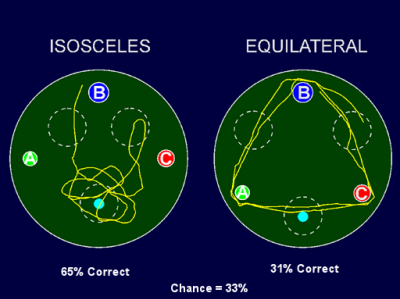 |
|
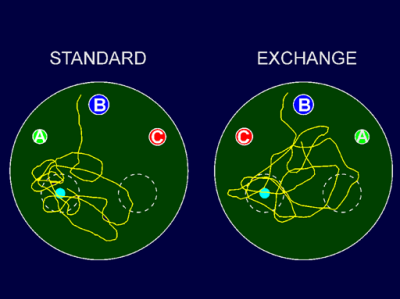 |
Figure 10. Benhamou and Poucet's swimming pool task. (Thanks to
Simon Benhamou for providing this picture.)
|
|
Figure 11. Landmarks vs. Configuration. (Thanks to Simon Benhamou for providing this picture.)
|
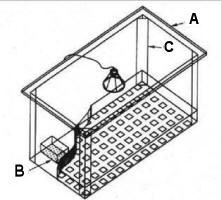 |
Figure 12. Apparatus used by Vallortigara et
al., 1990. a: One-way glass over the top of the
arena. b: Food box. c: Panel in the corner,
used in many of the experiments from Vallortigara's
group.
(Thanks to Giorgio Vallortigara for supplying this
and the following five pictures.) |
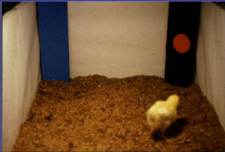 |
Figure 15. Examples of panels in the corner, with a chick at work on the
task.
|
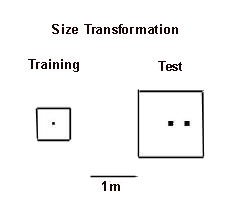 |
Figure 18. Size transformation test.
|
Vallortigara, Zanforlin, and Pasti (1990) tested chicks
in the reference memory paradigm, and found that the birds used
both geometric and featural properties readily. Figure 12 shows
a schematic drawing of their rectangular test apparatus. In all
the experiments, the food remained in one corner throughout
training. The animals were disoriented between trials, so that
they had to use cues within the arena to orient (see Figures
13-15).
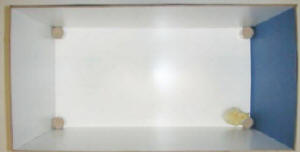 |
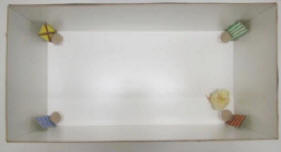 |
Figure 13. Overhead view of
a test situation. The blue wall is a featural cue, the
rectangular shape a geometric cue. |
Figure 14. Overhead view of
another test situation with panels in the corners serving as featural cues.
|
When features and geometry were provided (Figure
16,
left),
the chicks were mostly correct (results not shown). The chicks
showed no systematic rotational errors, unlike rats, young
children, and rhesus monkeys. This meant that they used featural cues. That the chicks learned geometry as well is shown on the
right (Figure 16), when all featural cues were removed for a test. The
chicks concentrated their searching in the correct corner
and the diagonal opposite, the rotational error. Without
featural cues, it is in principle impossible to distinguish
these two locations. Note that having the featural cues
during training did not overshadow the learning of geometric
cues. This is a theme found in most studies. See the
cue competition section.
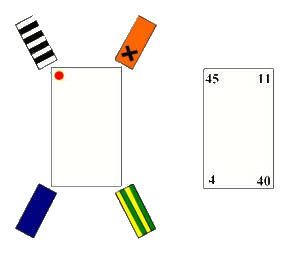 |
|
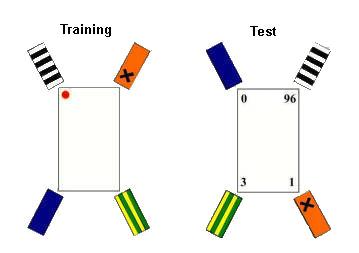 |
Figure 16. Results from Vallortigara et al., 1990.
|
|
Figure 17. Results of featural/configuration conflict test from
Vallortigara et al., 1990.
|
In the test shown in Figure 17, featural and geometric
cues were put in conflict. This was done by moving the nearest
feature, a beacon at the target, to a geometrically different
corner on a test (right, Figure 17). Chicks overwhelmingly opted
to 'go with' the beacon. Results of transformations of the
size of the training arena came later (Tommasi, Vallortigara, &
Zanforlin, 1997; Tommasi & Vallortigara, 2000). Chicks were
trained to search at the center of a square space. When the
square was expanded on a test, chicks showed tendencies to both
search at the center and to search at the correct absolute
distance from a wall (Figure 18). This suggests that both absolute and
relative distances, two kinds of geometric cues, are encoded.
Information on the neurophysiology underlying the
encoding of geometric and featural cues is given in the
neurophysiological section.
The results from pigeons resemble those from chicks (Kelly
& Spetch, 2001; Kelly, Spetch, & Heth, 1998). Pigeons, however, were also tested on a
vertically oriented computer monitor.
Figure 19A
shows an overhead view of an 'open field'
arena used by Kelly et al. (1998). Panels in
the corner served as featural cues, with the
food always at one of the corners (reference
memory task). The birds were disoriented
between trials.
Aside from two-dimensional panels, three-dimensional
objects in the corners were also used as featural cues
(Figure 19B). The results were similar in
the two cases. In the standard task, the
birds were nearly perfect; they did not make
systematic rotational errors. Thus, pigeons
use features in the relocation task. Systematic rotational errors
did appear when the features were degraded
in any of the manipulations that Kelly et
al. tried.
Removing the colors of features by making them
all the same color.
Removing the shapes of the features by making
them all identical in shape.
Removing the feature at the target and the
diagonal opposite.
Some birds were also tested with no features (Figure 19C). Identical landmarks in the corners make them non-diagnostic for locating the food.
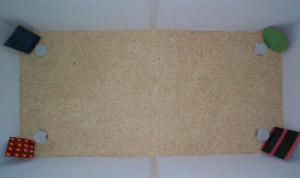 |
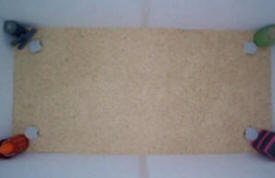 |
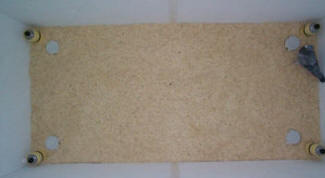 |
Figure 19A.
Kelly et al. apparatus. (Thanks to Debbie
Kelly for the pictures in this section.) |
Figure 19B. Kelly et al. apparatus with object cues.
|
Figure 19C.
Kelly et al. apparatus with no featural
cues.
|
Birds might be trained
without features, or trained with features
and then tested without features. In both
cases, the pigeons used the geometric cues
of the rectangle. They searched mostly at
the correct corner or its diagonal opposite.
Thus, as in chicks, having features during
training did not overshadow the learning of
geometry.
More on overshadowing in the
section on cue competition.
Pigeons were also tested with size transformations of the
training arena (Kelly & Spetch, 2001, Figures 20, 21). Figure 20 shows a smaller test
arena made by blocking off a part of the
full arena. The long wall here is equal in
absolute size to the short wall in the
training arena. Pigeons responded according to the shape. In this
example, they preferred to search at a corner with a shorter
wall to the right and a longer wall to the left, even though in
the test space, the absolute lengths of walls did not match
those in the training space.
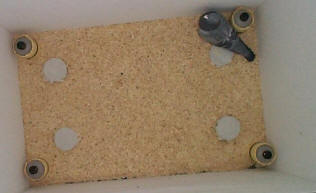 |
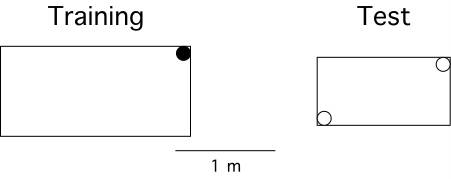 |
Figure 20. Kelly & Spetch (2001) apparatus (small version).
|
Figure 21. Kelly et al. size transformation
experiment.
|
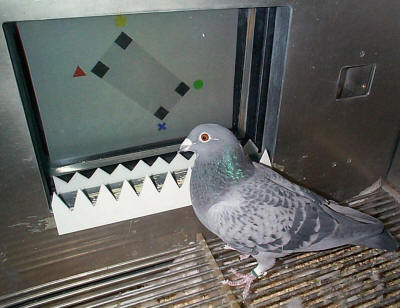 |
Figure 22. Stimulus and
subject in Kelly & Spetch's (2004b)
experiment. |
Touchscreen study on pigeons.
Pigeons have also been tested on a
vertically oriented computer monitor (Kelly
& Spetch, 2004b). In Figure 22, a bird
is shown facing
a schematic rectangle on the monitor of a computer. The task is
much like the typical arena task; one corner of the schematic
rectangle always leads to food when pecked. Pecks on the screen
are picked up by a touchscreen device. Food for correct responses is delivered to
one of the food magazines on each side of
the monitor.
Note that the orientation of
the rectangle can change from trial to
trial, and that all stimuli are vertically
presented. Both facts are important in
accounting for the results. In training, six
different orientations were used; two other
orientations (180 degrees apart) were saved
for transfer tests. Figure 23 shows a schematic example of the training
situation with both featural and geometric cues. Pigeons
learned this task readily. They cope
perfectly if the gray shading of the
rectangle was removed on the test. But other
manipulations led to worse but still
above chance performances:
Removing the color of features by making them all the same color.
Removing the shape of features by making them all the same shape.
Moving the configuration on the screen .
Removing the features at the target and the diagonal opposite, as shown in
Figure 24.
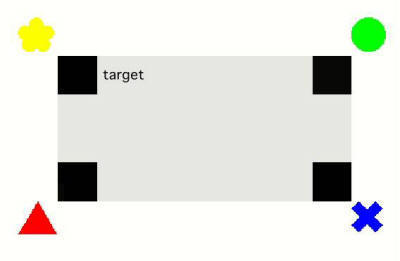 |
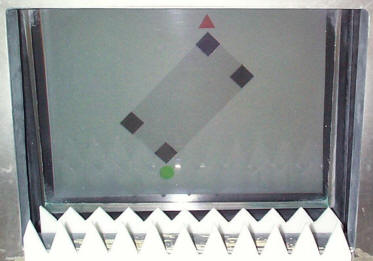 |
Figure 23. Stimulus properties in
Kelly & Spetch's (2004b) experiment.
|
Figure 24. A test with two of the features
removed.
|
Some
manipulations led to the appearance of
systematic rotational errors (Table 1).
When color information was removed by making
all symbols the same color (shape only),
when the feature at the target location and
its diagonal opposite were removed (distant
features), or when only a single feature at
an adjacent corner was provided on a test
(single feature), the percent of correct
choices decreased compared with control
tests. The errors, however, tended to be
rotational errors. If the errors were
distributed evenly, only one third of the
errors should have been rotational errors,
but the rotational errors in Table 1
exceed this chance value.
Test
|
% correct
|
% rot.
error
|
% other
errors
|
shape only
|
41.6
|
34.0
|
24.4
|
distant
features
|
59.6
|
24.9
|
15.5
|
single
feature
|
54.9
|
30.4
|
14.7
|
(on other
side of short wall)
|
|
|
|
|
Table 1. Results from Kelly & Spetch's (2004b) experiment. |
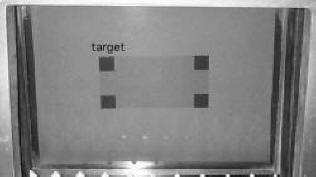 |
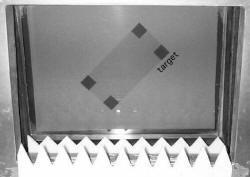 |
Figure 25. Pigeons were also trained and tested
without featural cues; this tests
whether they can learn to use
geometric cues alone.
|
Figure 26. Again, six
different orientations were used
in training, with two new
orientations (180 degrees apart)
saved for transfer tests. The
birds managed to learn geometry,
but a few characteristics of the
results are very interesting.
|
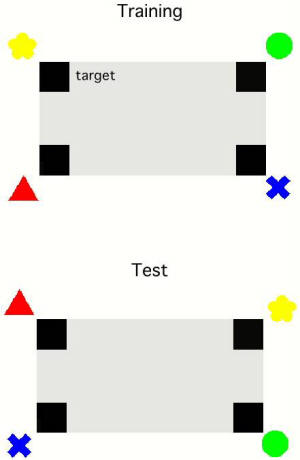 |
Figure 27. Featural and geometric cues
are put in conflict in the test shown in the
lower panel. At this stage, the birds had
learned both the featural task and the
geometric task. The birds' choices indicate
the use of both geometric and featural cues.
Thus, in this example, they chose the red,
green (geometrically correct) and yellow (featurally
correct) cues more often than the blue cross
(which is the only location that is both
geometrically and featurally wrong).
|
Again, six
different orientations were used
in training, with two new
orientations (180 degrees apart)
saved for transfer tests (Figures
25-27). The
birds managed to learn geometry,
but a few characteristics of the
results are very interesting.
Interesting results with geometric cues only.
When started on this task, learning was slow, significantly slower
than learning the featural task shown above.
Having learned the featural task first sped the learning of this task. Features, far from overshadowing the
learning of geometry, may have facilitated the learning of geometry.
Pigeons did not transfer at all to the new orientations. This
suggests that what they learned was orientation specific. A likely
explanation is that on a vertical surface, the orientation with respect
to gravity (i.e., up-down) defines a axis that is used for orientation.
Again, removing the gray shading of the rectangle did not affect
performance, while translating the rectangle led to a significant drop in
performance that was, however, still above chance.
Kelly and Spetch's (2004) data on human adults on the touchscreen task showed many similarities.
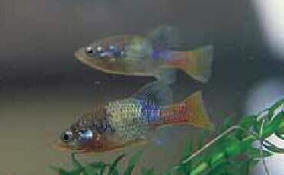 |
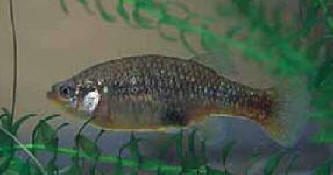 |
Figure 28.
Fish (Xenotoca eiseni) tested by Sovrano, Bisazza, and Vallortigara
(2002, 2003). Left: males; Right: female.
Thanks to Giorgio Vallortigara and Valeria Sovrano for
sending us pictures on their work,
featured in this section.
|
The task for the fish (Figure 28) was
aversively motivated. They were to swim out of
the rectangular arena, through one of the doors
in the corners, to join their peers. One of the
doors was always the correct one (the others
would not open). The arena was rotated from
trial to trial. Again, this was a reference
memory task. Figure 29A shows a condition in
which only geometric cues were available. The
fish learned this task, concentrating their
search at the correct corner and its diagonal
opposite.
Fish were also trained and tested
in arenas with featural cues, such as a blue
wall (Figure 29B) or panels in the corners
(Figures 29C). Figure 29D shows closer views of the panels in the corners. Fish learned to use the featural cues, a
colored wall or panels. They searched mostly
at the correct corners, and did not make
systematic rotational errors. In this way,
they resemble the birds, except that their
performance level was typically worse.
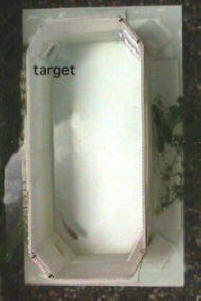 |
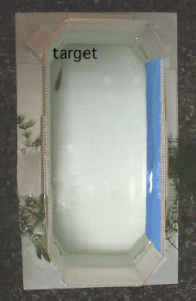 |
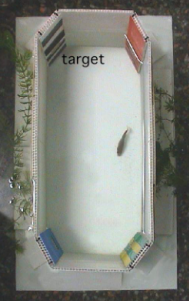 |
|
Figure 29A. Apparatus used in Sovrano, Bisazza, and Vallortigara
(2002, 2003)
|
Figure 29B. Apparatus showing featural cue.
|
Figure 29C. Apparatus showing featural cues in each corner. |
Figure 29D. View of featural
cues in corner panels.
|
When trained with features but tested
with features removed, the fish still
used geometric cues. In the example in Figure 30, they would search mostly at the
top left or bottom right. Thus, as with birds
and rats, learning to use features did not
overshadow the learning of geometry.
The fish were also presented with conflict tests (Figure
31). On
these tests (right), the panel at the target was
moved to a geometrically different corner,
resulting in a conflict between geometric and
beacon cues. The fish split their choices
between geometry and beacon. In this example,
they would search at the top left (geometrically
correct), top right (correct beacon), or bottom
right (geometrically correct). The results were
that the bottom left (geometrically and featurally wrong) was chosen the least.
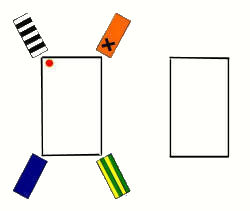 |
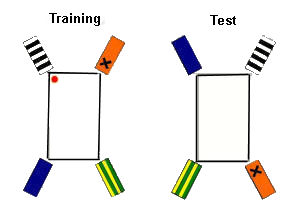 |
Figure 30. Schematic of
geometry-only test following
geometry & feature training.
Left: trained with features. Right:
tested without features.
|
Figure 31. Schematic of
conflict test.
|
Vargas, López, Salas, and
Thinus-Blanc (2004) tested goldfish (Carassius
auratus) in an escape task akin to the task
that Sovrano et al. (2002, 2003) used. The
goldfish were trained with geometric and
featural cues; featural cues were stripes on two
adjacent walls (Figure 32). Goldfish proved
adept at learning to use both geometric and featural cues.
On the test shown in Figure 33, the featural cues had been removed. The fish used
the geometric cues, searching mostly at the
correct corner or its diagonal opposite. On the
test shown in Figure 34, only featural cues were
provided; the arena had been transformed
into a square. Fish succeeded in the
task, choosing mostly the correct
corner.
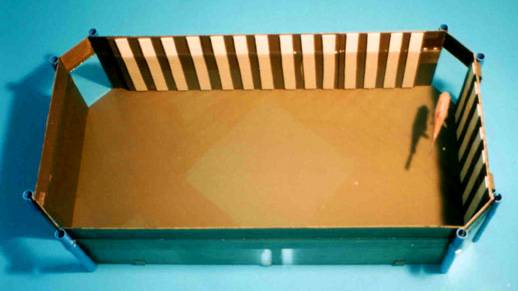 |
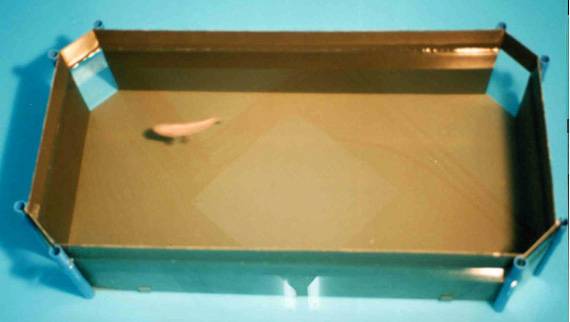 |
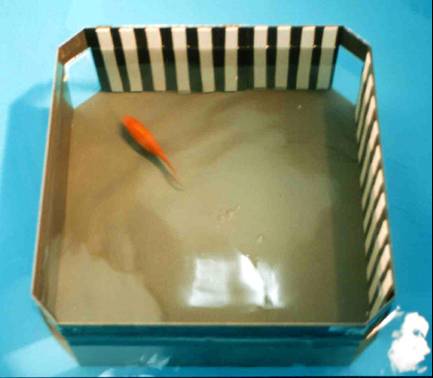 |
Figure 32. Rectangular
apparatus with featural cues. Thanks to Juan Pedro
Vargas for the photos of the
goldfish and apparatuses.
|
Figure 33. Rectangular
apparatus with featural cues
removed.
|
Figure 34. Square apparatus with featural cues.
|
Dissociation tests were also
conducted (Figure 35). On these tests, the stripes were
shifted in the manner shown (training situation
on left, test situation on right). On this test,
the fish were at chance choosing the four
corners. We explain the labels below. The results of a dissociation test depended on the
target location. With a target location at an intersection of
striped walls, the fish went with the featural
cues most (some 60%), chose the geometrically
correct corners next most, and chose the fourth
corner least (Figure 36).
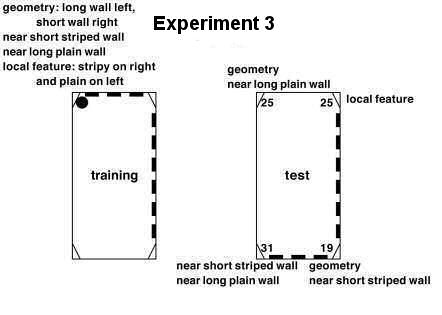 |
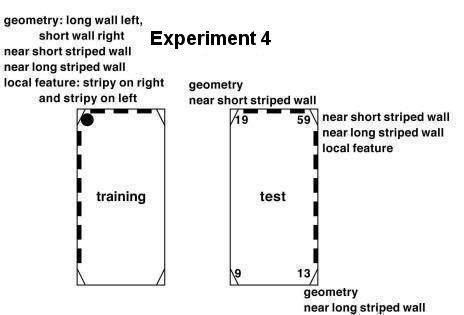 |
Figure 35. Results (percentages) and alternative
explanation of Experiment 3 of Vargas et al.
(2004).
|
Figure 36. Results (percentages) and alternative
explanation of Experiment 4 of Vargas et al.
(2004). |
Explaining dissociation tests.
Vargas et al.
interpreted the results from
dissociation tests as showing the
fish to use different strategies in
the two test situations. In the
first situation (their Experiment 3)
with the chance results, the fish
were said to use a map-like
strategy. In the second test
situation, in which the fish
preferred featural cues, they were
said to use a guidance strategy.
It seems problematic
to base an interpretation of
map-like strategy on chance results.
And the guidance strategy also does
not explain why geometrically
correct corners were chosen more
often than the fourth corner in the
second test situation.
As an
alternative explanation (Cheng, 2005), we
tallied up the likely properties that the
goldfish might attempt to match on a test
(left), and assumed that the fish would try to
match as many as possible. Geometry (long wall
to the left, short wall to the right) was one
property. A local feature to match consisted of
having stripy wall on the right and plain wall
on the left. (Panels were left out of
consideration because they are the same in every
corner.) A property of being near a wall of
particular length and decoration pattern (stripy
or plain), irrespective of which end of the wall
was nearest to the corner, was also considered a
property to match. The right panel shows that
these properties are distributed across the four
corners on the test in Experiment 3. One
reasonable prediction is that the fish would
search about equally at all four corners, a
prediction confirmed by the data.
In Experiment 4,
however, when the same set of
properties tallied on the left panel
are distributed among the different
corners on the test, we see that the
corner with the correct local
feature has the most properties, the
two geometrically correct corners
have the next most properties, while
the corner at the bottom left has
nothing to recommend it for
matching. The results reflect this
ordering.
Vargas
and López (2005) dispute this, on
the basis of other data, and matters
remain unclear.
Gouteux, Thinus-Blanc, and Vauclair (2001) tested rhesus
monkeys on reference memory tasks in a space (always of one
size) filled with different kinds of features across
experiments (Figure 37). Features might be a patch on the middle of a
wall or panels in the corners. The pattern of results
depended on the sizes of the features.
Small features:
With small features, monkeys used the geometric cues, but not the featural cues. Rotational errors were as frequent as
correct choices.
Large features:
With large features, monkeys used geometric and featural cues. Rotational
errors were systematic, so that on some trials, the
monkeys used only geometric cues. Figure 38 shows an example of an
experiment on rhesus monkeys from Gouteux et al.
(2001). On the left, results from tests with only
geometric cues, no featural cues. On the right,
performance when a whole short wall was made a
different color. Monkeys clearly chose the correct
corner more than the diagonal opposite (rotational
error). But the majority of errors consisted of
rotational errors.
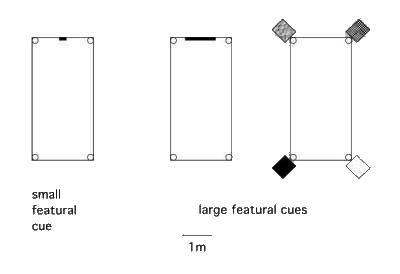 |
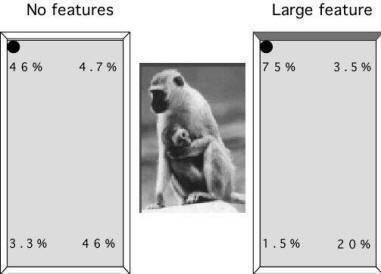 |
Figure 37. Large and small featural cues. |
Figure 38. Thanks to Catherine Thinus-Blanc for supplying
us the picture.
|
Hermer and Spelke
(1994, 1996) tested children of 18-24 months in a reference memory
paradigm, albeit one with only 4 trials. In all the experiments, the
hidden toy remained in one corner throughout training. The children
were disoriented between trials, so that they had to use cues within
the arena to orient. They found that the
children used geometric properties readily but did not use featural
properties. In later work, this lab found that featural cues began
to be used at 6 years of age and were virtually always used by
adults.
Figure 39 shows a
schematic drawing of their 4 by 6 foot
rectangular room. Figure 40 shows a photo of a room this size used by the Newcombe
lab. Figure 41 shows representative results from one of the Hermer-Spelke
experiments with children, followed by a
representative result with adults (Figure
42).
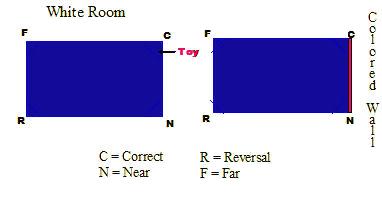 |
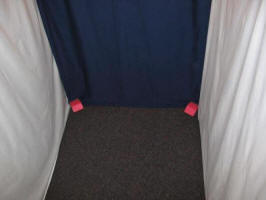 |
Figure 39. Hermer & Spelke (1994, 1996) experimental
conditions.
|
Figure 40. Overhead view of a small rectangular room with
identical boxes used to hide small toys.
|
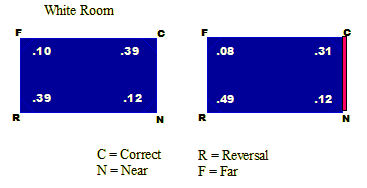 |
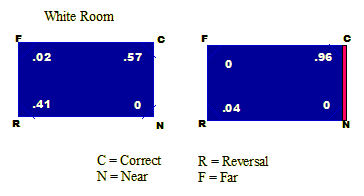 |
Figure 41. Hermer & Spelke: Search Rates for Toddlers.
|
Figure 42. Hermer & Spelke: Search Rates for Adults.
|
This pattern of data led the Spelke group to
propose the "Language-as-Bridge" hypothesis. As put by Hermer-Vazquez, Spelke & Katsnelson (1999, p.
34):
"[A]dults
may have a further system of representation that is uniquely
human and that emerges over the course of development. This
system may connect to many other systems of representation,
regardless of their domain-specific content. Its operation
may be governed by rules and principles allowing the
arbitrary combination of information from distinct,
domain-specific sources... The language faculty appears to
have all the right properties to serve as this uniquely
human combinatorial system of representation."
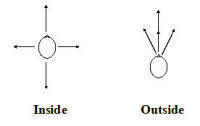 |
Figure 43. Perspectives inside and outside of a
space. |
More recent work has followed up both on young
children's sensitivity and on whether or not they use features
as well as geometry. Taking geometric sensitivity first, Huttenlocher and
Vasilyeva (2003) found that children could use geometry to
find a hidden object in enclosures of different shapes,
triangles as well as rectangles, both when inside and when
outside the space. However, they did better when inside the
space.
Children rarely surveyed the various locations before going
to the corner where they believed the object was hidden,
suggesting that they represent the entire space so that, no
matter where they face after disorientation, they know their
relation to the hiding corner. Using geometric information is easier when an organism is
inside a space than outside a space (Huttenlocher &
Vasilyeva, 2003). As shown in Figure 43,
one likely reason is that the distinctiveness of the space
is greater when inside.
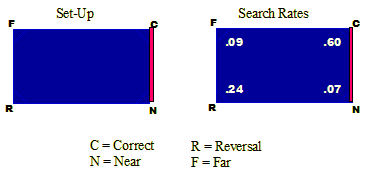 |
Figure 44. Learmonth, Newcombe, & Huttenlocher
(2001) experiment. |
Learmonth, Newcombe, & Huttenlocher (2001)
found that toddlers CAN use features to reorient,
as shown by the data in Figure 44. Searches were much more
likely in the correct corner than in the rotational
equivalent.
Room size is crucial to whether or not young children use featural cues. Learmonth et al. (2001) used an 8 by 12 foot
room, not a 4 by 6 foot room. Learmonth,
Nadel & Newcombe (2002, Experiment 1), using a room with
moveable walls, found that 4-year-olds did not use the
featural cue to reorient in a small room (C 35% vs R
41%) but did use it in a larger room (C 57% vs R 23%).
A small waist-high enclosure (Hermer-Spelke size) centered within
"large" room (Learmonth et al. size) gives sense of difference
in action possibilities between the two roomsthe
larger one is 4x the size of the smaller (Figures 45, 46).
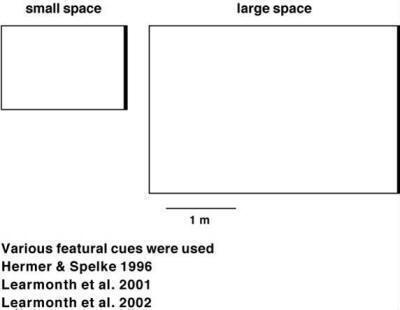 |
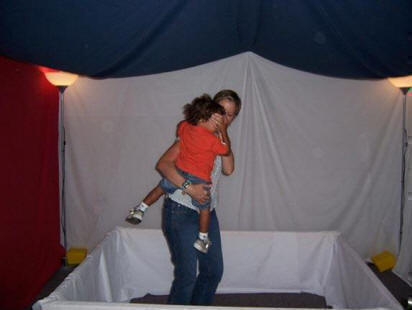 |
Figure 45. Comparison of spaces used in human
experiments.
|
Figure 46. Child being disoriented in small enclosure situated
within larger enclosure. |
Putting together the existing data, Cheng and Newcombe found the
developmental pattern shown in Figures 47 and 48:
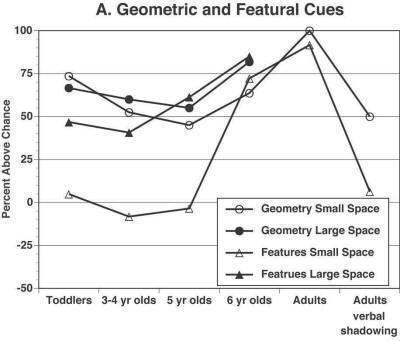 |
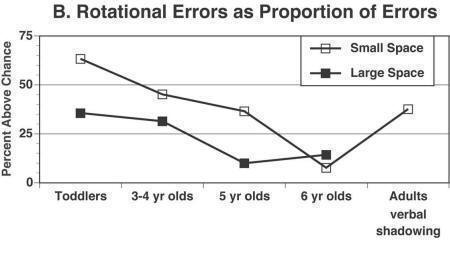
|
Figure 47. Geometric and Featural Cues.
|
Figure 48. Rotational Errors as Proportion of Errors. |
Looking at this developmental pattern undermines the language
hypothesis, which cannot explain:
Why toddlers succeed in the larger room.
Why there is improvement in rooms of both sizes before and
after hypothesized critical language acquisition.
Why room size makes a difference.
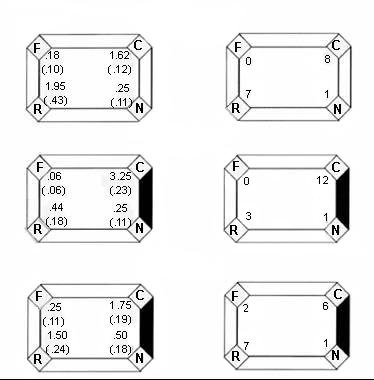 |
Figure 49. Results from Hermer-Vazquez et al. (1999). Adults
performed the task without verbal shadowing (top two
rows) or with verbal shadowing (bottom row). Left:
average number of each corner chosen out of 4 (SEM).
Right: first test only, pooled across subjects.
|
However, the fact
that adults doing verbal shadowing tasks do NOT use featural
cues (Figure 49) remains to be explained.
One new way to think about the roles of
geometric and featural information is that
the usage of each kind of information may vary with the
situation and with the learning history of the organism (or
other variables that determine individual differences). Consider
a recent experiment by Hartley, Trinkler, and Burgess (2004).
Using virtual reality, people were placed in arenas in which
they searched for targets. There were landmarks such as cliffs
located in the far distance. Figure 50 gives a sense of
the situation.
On some trials, the shape of the arena was
changed as shown in Figure 51. People's searches, as also shown
in the figure, were guided by a variety of kinds of spatial
information, including fixed distance to walls, a ratio of
distances, and angles to corners of the arenas. The relative
reliance on these types of information varied, with absolute
distances more heavily weighted when distances were shorter, and
thus likely to be estimated with less uncertainty. In addition,
when the shape of the arena was altered, some people seemed to
try to continue to use geometry to find the target while others
seemed to rely on external features (such as the cliffs) for
orientation. How different kinds of information are utilized and
combined will likely be understood better in the near future as
we analyse these kinds
of situational and individual information more closely.
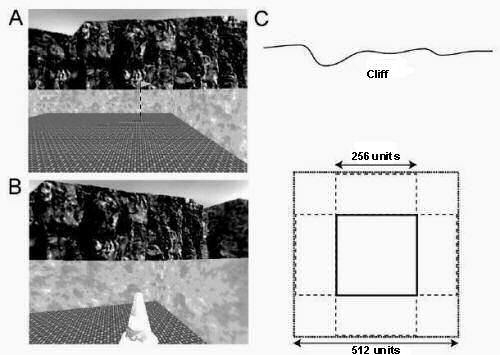 |
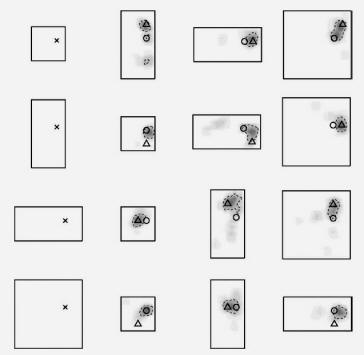 |
Figure 50. Stimuli (Panels A and B) and Layout
(Panel C) used in experiments of Hartley, Trinkler, and
Burgess (2004).
|
Figure 51. Results from test trials in experiments of
Hartley, Trinkler, and Burgess (2004).
|
In cue competition, the experience of one cue affects the
learning of a second cue adversely. In overshadowing, the
presence of an additional cue affects learning adversely; thus,
A is learned better alone than in AB compound. In blocking, a
previously learned cue affects learning adversely. Thus, if B is
learned in Phase 1, and AB is presented in Phase 2, A may not be
learned well. B is said to block the learning of A.
Cue competition is found in the case of learning to use landmark
objects to localize a target. Landmarks overshadow one another,
and a previously learned landmark can block the learning of a
new landmark (review: Chamizo, 2003). However, the picture is different when it comes to learning to
using geometric information.
In chicks (Tommasi &
Vallortigara, 2000), pigeons (Kelly et al., 1998) and rats
(Hayward et al., 2003; Pearce et al., 2001; Wall et al., 2004), a beacon (landmark right at the target) does not block
or overshadow the learning of geometric information.
Tommasi and Vallortigara
(2000, Figure 52, top left panel, top part) trained chicks to search
in the middle of a square. They either had a prominent beacon at the
center or not. The beacon was a potential cue competitor with
geometric cues. However, when tested with the geometric cues alone (without
the beacon), both groups performed similarly, giving no
evidence of the overshadowing of geometric cues by a beacon.
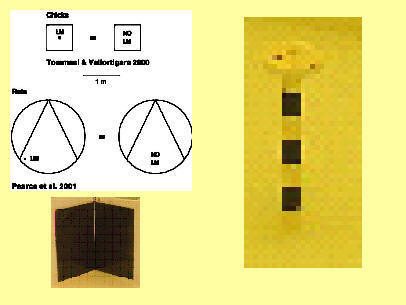 |
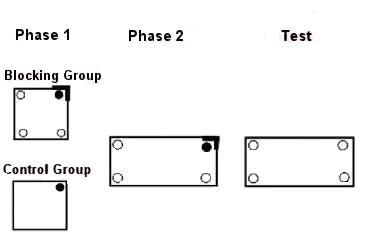 |
Figure 52. Top-left - The situations
used by Tommasi and Vallortigara (2000) and
Pearce et al. (2001). Bottom-left and
Right - Beacons used for rats (color photos thanks
to Anthony McGregor).
|
Figure 53. An experiment from Wall et al. (2004).
|
Pearce et al. (2001) and
Hayward et al. (2003) trained rats to find a corner of a swimming
pool, where a hidden platform was located for escaping the water. An
example is shown in the top left panel, bottom part of Figure 52. They found that
having a prominent beacon right at the target corner neither
overshadowed nor blocked the learning of geometric cues. The rats in
these situations with cue competition still managed to find the
target without the beacon present, as well as animals that learned
geometric cues without a beacon. A beacon failed to be a cue
competitor of geometric cues.
Rats in the blocking group learned to use a beacon to find
food in one of four corners in Phase 1 (Figure 53). Control rats had
only the food dish, without the beacon, in Phase 1. In Phase
2, geometric cues were introduced in the form of a
rectangular arena. Geometric cues were not as good a
predictor as the beacon, because it left a two-fold
ambiguity. Nevertheless, both groups showed equal
evidence of using geometric cues, because they made
rotational errors to similar extents. In the test with
geometric cues alone, both groups proved to use geometric
cues equally well. Thus, a prominent beacon did not block the learning of
geometry. Wall et al. concluded that beacons and geometry
are learned independently.
Since writing this section,
two studies have recently found cue competition with geometric cues,
in mountain chickadees (Gray, Bloomfield, Ferrey, Spetch, & Sturdy,
2005) and rats (Pearce, Graham, Good, Jones, & McGregor, 2006). Both
provided featural cues not as local beacons, but colors of entire
walls.
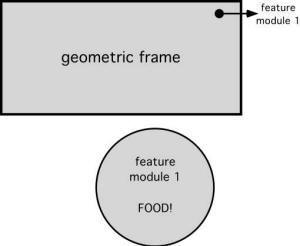 |
Figure 54. A single feature module for
encoding spatial information (Egerton
et al., 2000).
|
A check on recent citations reveals three different sources in the literature
on artificial intelligence that have cited Cheng's (1986)
paper. Two take the notion of encoding geometry seriously,
while one demonstrates how a robot may perform without
encoding geometry.
Egerton, Callaghan, & Chernett (2000) took the idea
of a geometric module or geometric frame to heart.
They suggest a division of labor in encoding spatial
information. A geometric frame or module encodes the
broad shape of the environment. On it are pointers
to feature modules. Feature modules tell about what
is to be expected at a particular place or region in
space. Figure 54 shows a single feature module,
but a functioning system would have a substantial
bank of features.
A key
point of this separation is to help solve what is
called the problem of perceptual aliasing. This
refers to the fact that a purely featural
description leaves much ambiguity. For example, the
description "door" might fit several places in one's
space. But a door at a particular region is
typically a
unique description. Having a global frame makes the
use of local information unambiguous. It saves the
system from having to encode detailed local
information.
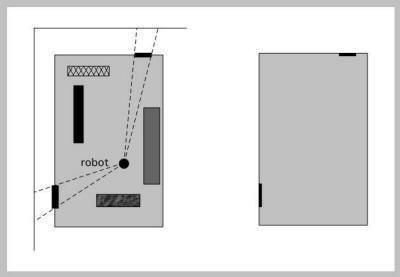 |
Figure 55. The Absolute Space Representation
(Yeap & Jefferies, 1999).
|
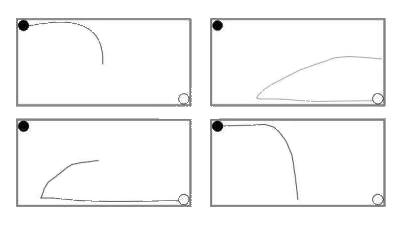 |
Figure 56. Models of robots problem solving
(Nolfi, 2002).
|
Another representation that encodes geometry is the Absolute
Space Representation (Yeap & Jefferies, 1999). Yeap
and Jefferies were concerned with early cognitive mapping,
which has the goal of getting a robot to build up a map of
the environment it is navigating through. They suggest the strategy of having the robot build
an early map of a local environment specifying where
boundaries (such as walls) and exits are. The idea is that
the robot should at first 'look past' furniture that clutter
a room (indicated in the left panel of Figure 55 by variously shaded
rectangles) and figure out where the outer boundaries are.
Such a frame with exits allows the robot to navigate through
its environment while it builds up more knowledge.
Robotics can benefit the study of animal behavior as well as
vice versa (Webb, 2000). The Absolute Space Representation
points out the importance of encoding exits. In the animal literature on geometry, the importance
of exits has not yet been tested. If exits are important,
then they might serve to disambiguate rotational symmetry,
whereas other objects of equivalent size might not. Testing
this hypothesis is straight forward, and deserves to be
done.
Nolfi (2002)'designed' reactive agents for solving Cheng's
(1986) task up to rotational ambiguity. A reactive agent has
no internal states, and reacts to a stimulus always in the
same way. "Design" is in quotes because Nolfi actually let
the systems evolve. Better solutions got to 'reproduce,' with some
variations, until a system evolved that can readily solve the task.
The left panels of Figure 56 illustrate a solution for solving the problem
starting from the middle, facing one of the walls. Basically, the
robot walks with a veer to the left, until it meets a wall. Then it
turns left and hugs the wall until it runs into a corner. If the
veer is within a certain range, the robot mostly reaches either the
correct corner (filled dot) or its diagonal opposite (open dot). The
right panels show a similar solution with the middle of one of the
sides (facing center) as starting points. Historically, these were
the positions used in Cheng (1986). Nolfi was not concerned to show that animals solve the
problem this way. Rather, he was demonstrating the powers of
reactive agents.
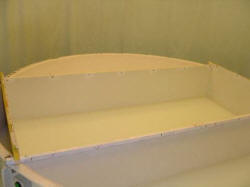 |
Figure 57. Pearce et al. (2004) apparatus. Thanks to Anthony McGregor for
the photo. |
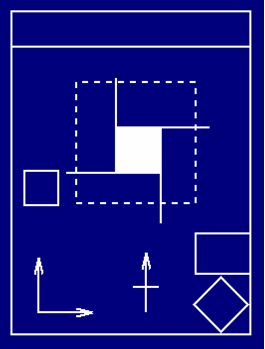 |
Figure 58: O'Keefe and Burgess (1996) apparatus. Thanks to
Neil Burgess for this picture. |
The hippocampus is well known for its role in spatial
navigation (O'Keefe & Nadel, 1978). It is beyond the scope
of this chapter to review the literature on the hippocampus
and space (review: Jeffery, 2003). But we present here some
results concerning the encoding of geometric properties.
Hippocampal lesions in rats reduce
rats' ability to choose the geometrically correct
corners of a rectangular pool (Pearce et al., 2004).
The pool (Figure 57) is surrounded by a circle of
curtains, and would be filled with water during
experimentation.
O'Keefe and Burgess (1996) recorded from the
rat's hippocampus as the rat wandered in a rectangular
space. The rat was oriented and had external cues to
direction. It searched in the white rectangle, whose shape
can be changed by shifting the walls appropriately (Figure
58).
Hippocampal place cells (O'Keefe &
Nadel, 1978; Figure 59) are typically found in the hippocampus proper.
They fire preferentially when a rat is at a particular place
in space.
In Figure
59, the 'training' space was the
vertical rectangle on the bottom left of each panel. The rat was
then transferred to transformed spaces (the other diagrams) and
the firing rate of each cell recorded. What the pattern of
results from 28 cells show (see also Hartley, Burgess, Lever, Cacucci, & O'Keefe, 2000) is that these hippocampal cells are
sensitive to both absolute distances from walls, and to relative
distances, varying according to circumstance. Below in this
section, we present the group's model explaining this.
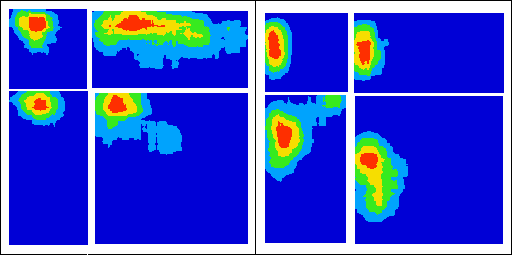 |
Figure 59. Adapted from Figure 1, O'Keefe & Burgess, 1996. Thanks to
Neil Burgess for the picture. |
The 'performance' of 35 recorded place cells are shown in Figure
60. These firing rates are obtained independent of the direction that
the rat is facing.
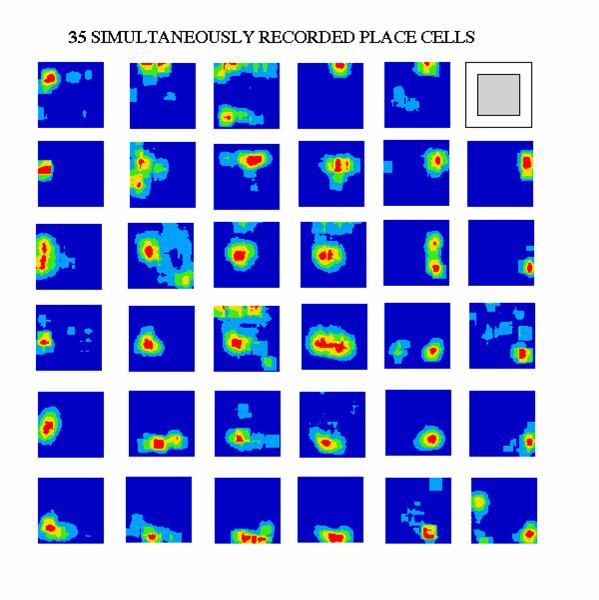 |
Figure 60. Adapted from Burgess et al. (2000, Figure 1). Thanks
to Neil Burgess for the picture. |
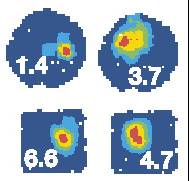 |
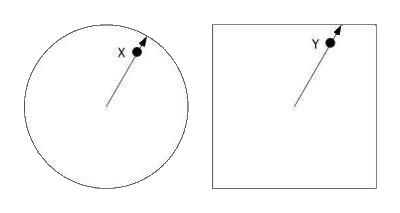 |
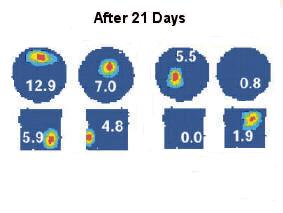 |
Figure 61. Taken from Lever et al. (2002, Figure 1).
|
Figure 62. Place cells firing in corresponding
locations in different shapes.
|
Figure 63. Taken from Figure 3 of Lever et al. (2002).
|
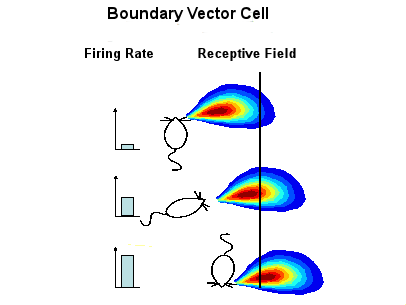 |
Figure 64. Adapted from Hartley et al. (2000,
Figure 1). Thanks to Neil Burgess for the picture.
|
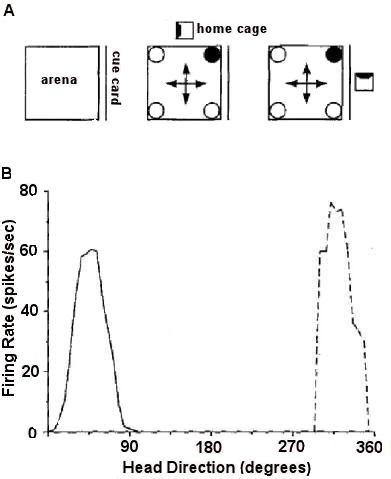 |
Figure 65. Adapted from
Golob et al. (2001),
Figures 1 and 2. |
The shape of the 'training' space can be more radically
transformed, and the hippocampal cells will still show
systematic firing patterns (Lever,
Wills, Cacucci, Burgess, & O'Keefe, 2002; Figure 61). For example, a square
may be turned into a circle. Often, cells would fire at
corresponding locations in the two spaces (Figure 62).
Correspondence is determined as follows. If X is
the place of maximal firing in the training space (left), then
Y, the place of maximal firing in the transfer space (right)
lies in the same direction from the center, at the same
proportion of distance from the center to the edge.
Over many days of repeated exposure to both
spaces, however, the 'behavior' of the cell in the two spaces
may change. After 21 days, a cell might respond in one space but
not the other (Figure 63, right), or might respond at different,
non-corresponding places in the two spaces (Figure 63, left).
To model the performance of place cells, the
existence of boundary vector cells are posited (Burgess et al.,
2000; Hartley et al., 2000; Figure 64). These cells, which have not to date
been found, respond when the rat is at a particular distance and
direction from a surface (the black line), independent of the
direction that the rat is facing. The boundary vector cells are
upstream from the place cells, and a conjunction of boundary
vector cells determine the performance of a place cell. This discussion of place cells in the rat
hippocampus suggests that these cells perhaps play a
role in encoding local geometry, vectors to surfaces,
and not the global orientations that determine which
direction is which.
Other cells in
the hippocampal complex, called head direction cells,
are sensitive to the direction that the rat's head is
facing, irrespective of the place that the rat is
currently at (review: Dudchenko, 2003). These cells are
typically found outside the hippocampus proper, in
diverse areas ranging from the lateral dorsal thalamus
to the dorsal tegmental nucleus. As these cells appear
to encode direction, we may wonder whether their
performance has something to do with encoding geometry
and with rotational errors.
Golob, Stackman,
Wong, and Taube (2001) probed this question. Head
direction cells were recorded while a thirsty rat was in
a square arena (Figure 65A). The rat was not
disoriented, but circular curtains surrounded the arena.
Its home cage might change locations on different
trials, as illustrated in Figure 65A. A cue card on one
wall provided directional cues. The rat had the
behavioral task of finding one constant corner at which
water was available; this was thus a reference memory
task. The performance of a head direction cell was
generally consistent, but on occasional trials, its
preferred direction might shift by some multiple of 90o,
as the dashed curve in Figure 65 shows.
The question
that arises is whether these shifts in the performance
of head direction cells predict behavioral errors on the
part of the rats. Across a number of experiments, the
answer was a clear no. Golob et al. (2001) found no significant
relations between the preferred direction of head
direction cells and behavior.
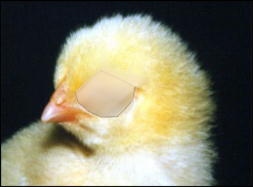 |
Figure 66. Thanks to Giorgio Vallortigara for the pictures on
chicks in this section.
|
Many birds show many hemispheric specializations
for behavior. Visual input to the bird brain is highly
lateralized. By covering one eye, as in Figure 66,
information is effectively restricted to half the brain. With
the left eye covered, this chick is a left-brained animal
visually. Vallortigara, Pagni, and Sovrano (2004) used eye
covering to test the role of the hemispheres in encoding geometric
and featural cues. In this experiment, chicks were trained with
geometric and featural cues, but tested for their use of geometric
information. Binocular and left-eyed (right-brained) chicks did
fine, while right-eyed (left-brained) chicks failed (Figure 67).
The experiment in Figure 68 tested the use of featural cues.
In the tests, geometric cues were removed by making the space
square. Both left-eyed and right-eyed chicks did fine,
indicating that both hemispheres encode featural cues. In the
experiment shown in Figure 69, both the training and test situations had
only geometric cues. Left-eyed (right-brained) chicks again did fine.
Right-eyed (left-brained) chicks were marginal (did not quite beat
chance statistically, at p = 0.06).
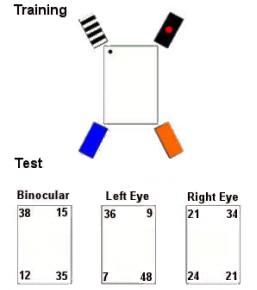 |
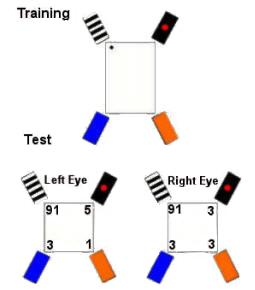 |
Figure 67. Procedure and results from Vallortigara, Pagni, and Sovrano
(2004). |
Figure 68. Testing featural cues by
removing geometric cues from
Vallortigara, Pagni, and Sovrano (2004).
|
The study shows that the left hemisphere
encodes only features, while the right hemisphere
encodes both features and geometry. Tommasi, Gagliardo,
Andrew, and Vallortigara (2003) used lesions of the
right or left hippocampus of chicks in the relocation
task. They came to similar conclusions. The left
hippocampus encodes only featural information, while the
right hippocampus encodes geometric and featural
information.
The role of the hippocampus in geometric encoding
has also been studied in homing pigeons (Vargas et al., 2004). Normal pigeons and birds with their hippocampus lesioned
(bilaterally) were trained in a space containing both featural
and geometric cues (Figures 70 and 71). Both learned the task, but interestingly,
birds without hippocampus reached criterion faster. It seems
that learning geometry (in birds with hippocampus) is
obligatory, even when it does not help. Even though geometric
information is ambiguous and may cause rotational errors, it is
nevertheless learned. This point is also clear from the studies
on cue competition.
When featural cues (a red wall) were removed on a
geometry test, control birds used the geometric cues, whereas
birds with their hippocampal formation lesioned failed (Figure
71). When geometric cues were removed by making the
search space square on a feature test, both control and lesioned
birds succeeded (Figure 72). When geometric cues were put in conflict with featural cues, by making a long wall rather than a short wall red,
control birds went with the geometry whereas lesioned birds went
with the features (Figure 73).
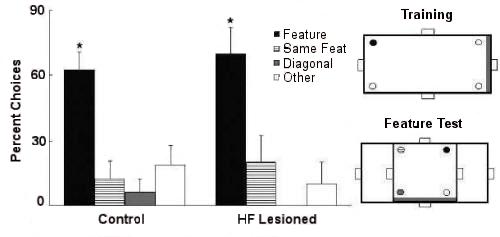 |
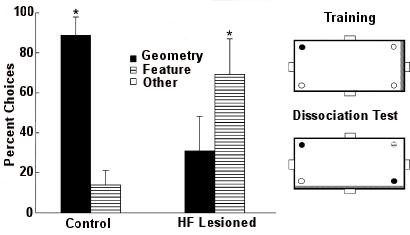 |
Figure 72. Feature Tests. Adapted from Vargas et al. (2004, Figure 5). |
Figure 73. Dissociation Test. Adapted from Vargas et al. (2004, Figure 6). |
Parahippocampal place area?
It has been proposed that the geometric
layout of the environment is constructed in a specific area
of the human brain, namely the posterior tip of the
parahippocampal gyrus and adjacent regions of the fusiform
gyrus (Epstein & Kanwisher, 1998; Epstein, DeYoe, Press,
Rosen, & Kanwisher, 2001; Epstein, Graham, & Downing, 2003;
Epstein, Harris, Stanley, & Kanwisher, 1999, Figure 74). This area has
been termed the Parahippocampal Place Area (PPA).
Human imaging evidence.
Perception of scenes led to differential
activation in the PPA, relative to perception of faces,
objects, or houses (Epstein & Kanwisher, 1998, Figure 75), even though
the subjects were not required to perform any tasks.
More evidence on PPA and geometry.
Coherent geometric structure seemed vital to PPA activation
because fractured and rearranged versions of the bare rooms did
not elicit a response. Further, activity in the PPA is not further increased when
people feel as if they are moving in a scene, suggesting that it
is more involved in geometric analysis than in planning routes
or monitoring locomotion (Epstein et al., 1999).
PPA seems viewpoint invariant.
Imaging of PPA activity while people looked at scenes such
as those in Figure 76 showed that a change in viewpoint (middle
row) led to as much activity as a complete change in
place (at bottom) with both elevated relative to
no-change control (at top).
Doubts about the PPA as possible substrate for
geometric module.
There are alternative interpretations of the role
of human parahippocampal cortex (Maguire, Burgess, et al. ,
1998; Maguire, Frith, et al., 1998; see also Maguire, Burgess, &
O'Keefe, 1999; O'Keefe et al., 1999). They have imaged humans performing
a variety of tasks such as navigating around a virtual town (see Figure
77). This group argues that the parahippocampal area
is especially involved in locating objects in allocentric space.
In fact, Burgess and O'Keefe (2003) suggest that the human
parahippocampus is better termed a Spatial Scene Area than a
Place Area.
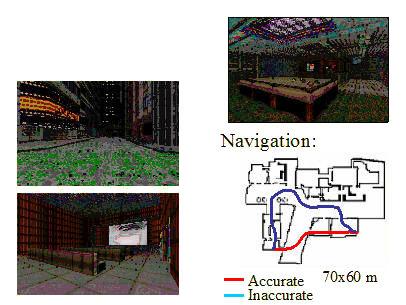 |
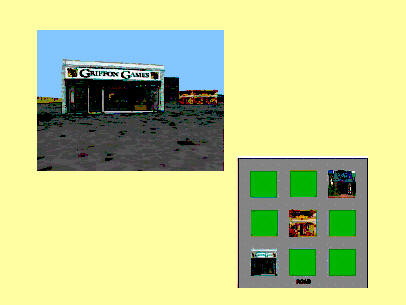 |
Figure 77. Virtual Reality Town for
Testing Spatial and Episodic Memory. Used by
Burgess and colleagues. Photo courtesy of Neil Burgess. |
Figure 78. Example stimulus (left) and stimulus layout (right)
in Ekstrom et al. (2003). Photo coutesy of Michael
Kahana. |
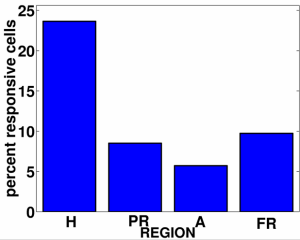 |
Figure 79. Ekstrom et al. data. Courtesy of
Michael Kahana.
|
Place cells in the hippocampus?
Ekstrom et al. (2003) conducted the first single-cell
investigation of humans -- 7 patients with pharmacologically
intractable epilepsy who were being observed with intra-cranial
electrodes participated as taxi drivers in a VR navigation game
(Figure 78). They picked up
passengers and took them to one of nine distinctive buildings
arranged in a grid.
Cells were categorized as place cells if
they responded when the patient was in a particular location in the
grid, independent of what view was on the screen or what goal was being
sought. Similarly cells could be categorized as view cells or as goal
cells if they responded to particular views or goals but were not
affected by the other two types of information or by interactions. Place
cells predominated in the hippocampus (as shown Figure 79, courtesy of
Michael Kahana)
and view cells in the parahippocampus.
Given the amount of evidence showing that
all vertebrate animals tested so far use the geometric
properties of space, surprisingly little has addressed
how they use geometry or what aspects of geometry play a
role. The only prominent theory was formulated by
Gallistel (1990, Chapter 6). Gallistel theorized that rats
encoded and matched the principal axes of space for
determining which direction is which in space. The
direction determining is done by matching something far
less and far simpler than the entire shape.
The major (long arrow) and minor (short
arrow) principal axes of a rectangle are shown in Figure
80. A
principal axis goes through the centroid of the space. A
centroid is the center of mass. We have assumed in this
diagram that the density of 'mass' in the space is
uniform, so that the centroid is at the center. But by
postulating non-uniform 'subjective density' of space, the centroid might be displaced from the center.
The major (first) principal axis has
these properties:
Mechanically, if the space spins
around the axis, it would have the minimum angular
momentum.
Statistically, the sum of squares of
perpendicular distances of points in space to the
axis is minimized.
Figure 81 shows that it is the
perpendicular distances to the axis that are minimized,
not distances to the principal axis along some arbitrary
axis (such as the y-axis, as in statistical regression
analyses). The minor (second) principal axis is
perpendicular to the major axis, but we will not be
concerned with it.
The principal axis is a computationally
shorthand way of coding global directions without having
to encode all the details of the overall shape. It is
simpler than the geometric module in Cheng (1986) and
Egerton et al. (2000), or the Absolute Space
Representation of Yeap and Jefferies (1999). It is a
parameter that can be matched with a deterministic
routine, without the use of iterative trial and error.
The matching does not 'explode' in complexity as the
space becomes more detailed and complicated in shape.
For over a decade, nothing addressed
Gallistel's (1990) theory. Then two papers were
published in 2004 that claimed to rule out any global
geometric representations, including presumably the
theory of principal axes. Pearce, Good, Jones, and McGregor (2004)
trained and tested rats in a swimming pool task, with
the target escape location at a corner (Figures 82, 83, left). The pool
was ringed by curtains and rotated from trial to trial.
With no featural cues, the rats of course made
rotational errors, as often as correct choices. In a crucial test, the shape of the pool
was changed to a kite shape (Figures 82, 83, right). This changes
Euclidean properties, so that the global shapes of the
training and test spaces could not be matched.
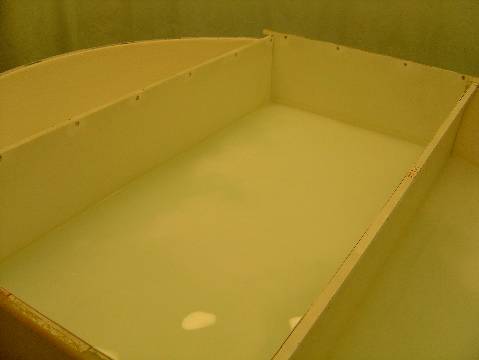 |
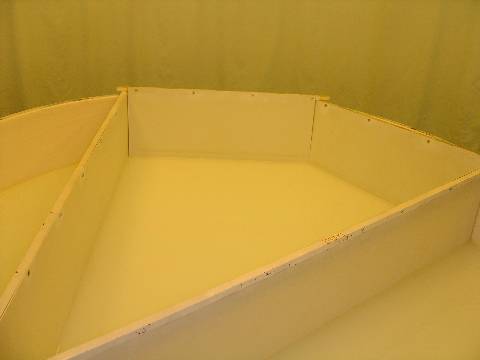 |
Figure 83. The rectangle (left) and
kite (right) pools used in the Pearce, et al.
(2004) experiments. Both pools would be filled with
water during experimentation. Thanks to Anthony McGregor
for these photos.
|
Can a formulation in terms of the
principal axis of space explain this behavior?
The answer is a definite yes
(Cheng & Gallistel, 2005). In Figure
84, the first principal axis of the test
space is shown. In dotted lines, the training
situation is superimposed, lined up at middle of
the principal axis, and in the direction of the
principal axis. Instantly, we see that the rats'
chosen corners are near the ones defined in the
training space. One needs to define distance
(nearness) properly to favor the top right
corner of the kite over the obtuse angle at the
top. This is readily done by assuming that the
rat is looking for something like: the end of
the principal axis and as far to the right as
possible. In the scheme shown in Figure 85, searching at the apex is
akin to a rotational error. When the rat heads down
toward the apex end of the kite, it has only one corner
to go to.
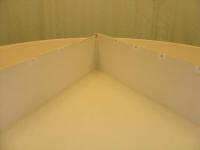 |
Figure 85. Thanks to Anthony McGregor for this
photo.
|
Another study on chicks in an appetitive
food finding task had the birds searching for food in
one of four containers placed in the corners of a
parallelogram. (Tommasi & Polli, 2004). The filled and
open dots in Figure 86 show the target locations
of two different groups of chicks. It is crucial to show
the results of both locations because they produced
important differences.
In the training situation, the chicks,
without any featural cues, of course made rotational
errors and chose the diagonal opposite to the target as
often as the target. In the figures here, this fact is
not shown. Figure 87 shows where the chicks
searched in transformed spaces. Again, the chicks make
rotational errors (not illustrated). They searched at
levels as high as in the training space. The rectangle (left) preserved the
lengths of walls, but altered the angles of corners. The
authors' interpretation is that the chicks encoded the
relative lengths of walls. The rhombus (middle) preserved the angles
at the corners, but altered the lengths of walls and
their ratios to one another. The authors' interpretation
is that the chicks encoded the corner angle.
A reflection of the training space
(right) produced the most interesting results. In this
space, corner angle and relative lengths of walls were
put in conflict. Where the corner angle matched the
training situation, the relative lengths were incorrect.
For example, for the black-dot group, trained at an
acute angle, the long wall was to the right of the short
wall in the test space, but to the left of the short
wall in the training space. Thus, the acute-angle group
(black dots) matched corner angle. The obtuse-angle
group (open circle), who chose the same acute-angled
corners, matched the relative lengths of walls rather
than corner angle. The authors' interpretation is that
an acute angle was more salient than an obtuse angle.
The chicks were basically matching on the most salient
cue.
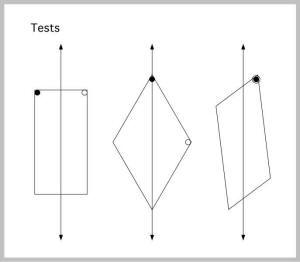 |
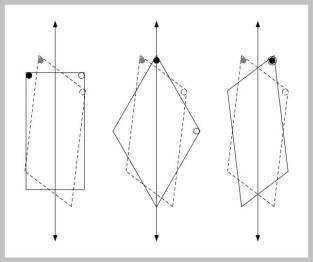 |
Figure 87. Tommasi and Polli (2004) experiment - Tests.
|
Figure 88. Superimposition of training and testing apparatus with
principal axis shown.
|
Again, can the pattern of results be
explained by reference to the principal axis of space,
something that the authors rejected? Again, the answer is a definite yes
(Cheng & Gallistel, in press). In Figure 88, the ghost
of the training space (in dashed lines) is again
superimposed on each of the test spaces, with the target
locations shown in gray for the acute-angle group (black
dots in the test spaces), and in open circles for the
obtuse angle group (open circles in the test spaces).
Again, test spaces and training space are lined up at
the principal axis. If the chicks extracted the
principal axis of space, and searched at the nearest
location according to the training situation, the figure
shows that they would search at the observed places that
they chose in the test situations. In this case, other
than going to a food bowl, there is no need to encode
any other local features, such as the angle of a corner.
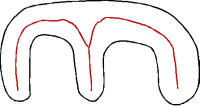 |
Figure 89. An M-shape, with some axes of symmetry drawn through it
in red by free hand.
|
Cheng (2005) suggests
that principal axes may not be the best scheme for capturing
global shape information. The trouble is that a principal axis
is always a straight line, no matter what the shape; it really
does not tell us anything about the shape. The M-shape in Figure
89 has a roughly horizontal straight line as a principal axis,
just as a horizontally oriented rectangle would.
In Figure 89, we have
also drawn in red, by free hand and approximately, a set of axes
of symmetry. These bend with the curvature of the shape and
capture regional symmetries, roughly bisecting the nearest
walls. A glance at the red axes shows that they capture the M of
the shape. Such axes of symmetry, a variety of them, can be
formally defined (see Leyton, 1992, ch. 6).
Another shape-capturing
scheme is the medial axis scheme (Fritsch, Pizer, Morse, Eberly,
& Liu, 1994; Pizer, Fritsch, Yushkevich, Johnson, & Chaney,
1999), designed for the purpose of medical imaging. In brief
this kind of scheme draws a stick-figure trunk and branch
structure to capture the gist of the shape. The trunk goes
through the middle of the space, and the branches indicate
distances to the boundary. Substructures, such as the middle
finger of the M in Figure 89 would have their own skeletons,
and be linked to the 'parent' shape. The scheme thus also
captures something of the structure of the shape.
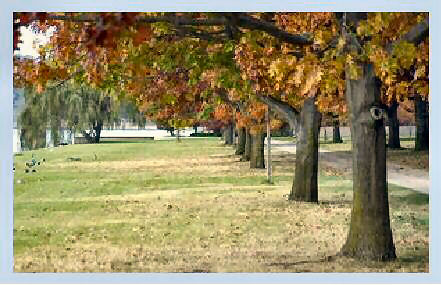 |
Figure 90. Axes in a natural scene?
|
A priori, it is likely that some kind of
global representation of space is used for navigation.
The global representation helps the animal to use the
right local cues. The problem with local cues is that
there are too many of them (the problem of perceptual
aliasing, see section on artificial intelligence). The
world has too many trees and stones.
Having a sketchy global representation
(such as representing key axes) helps the animal limit
the amount of local information that has to be encoded.
As an example, imagine encoding enough information about
a tree shown in Figure 90 to distinguish it from
every other tree in this part of a park. The problem
taxes the best 'featural' systems, and explodes in
complexity with the number of trees. If, however, a
global representation limits the search to a small
region, then the number of trees are limited, and the
problem does not explode in complexity. Judiciously
combining global and local information might make the
task tractable at many scales.
These studies are important in forging
ahead with methods for testing theories; they provide
good methodological models.
Since we wrote this,
recent work from Pearce's lab have continued the discussion
about the nature of geometric encoding (Graham, Good,
McGregor, & Pearce, 2006; McGregor, Jones, Good, & Pearce,
2006; Pearce et al., 2006). Far more research using many
transformations is needed to constrain theories of
geometric encoding.
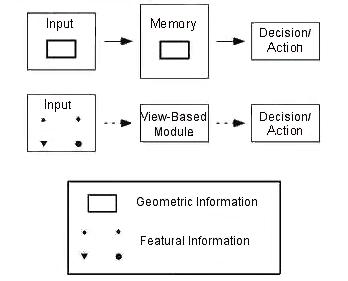 |
Figure 91. Impenetrable Module. Strong Modularity.
|
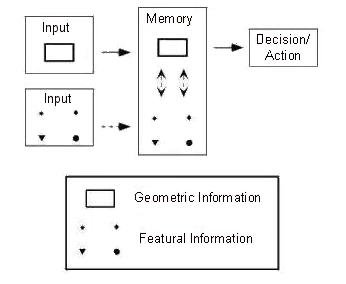 |
Figure 92.
Modular Memorial
Subsystems. Modular Subcomponents. |
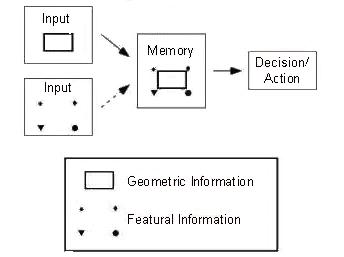 |
Figure 93. Modular Input Systems Only. Integrated. |
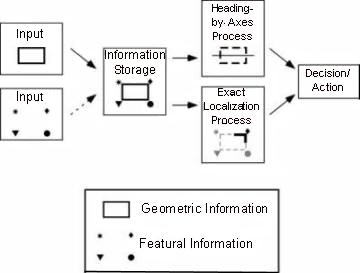 |
Figure 94. Theory proposed by Cheng (2005) incorporating
elements of all three versions of central
modularity. |
Figures 91, 92, and 93 (adapted from Figure 3 of Cheng and Newcombe, 2005), show 3 views of modularity that we can
identify. Doubtless there are other versions. These views
concern modularity in the central system(s) that represent
information. We consider below a notion of modularity at
output.
In
all three views, we have characterized the input processes
as modular. Thus, we assume that different visual channels
handle and compute geometric and featural properties, a view
consistent with Fodor (1983). In fact, visual systems are
far more modular than the two boxes shown in the following
three pictures (Marr, 1982).
In a strong view of modularity (Wang
& Spelke, 2002, 2003), geometric information goes
through an impenetrable module that handles only
geometric information. "Impenetrable" means that the
module does not admit any other kind of information. Featural information, if it is used,
is handled by other modular systems. We have listed
a view-based module as one example. Thus, the
systems run parallel through central processing to
action.
Some processes use only the geometric
route. In Wang and Spelke (2002), it was the process
of determining direction after disorientation. In
Wang and Spelke (2003), it was either the process of
determining direction or the process of locating a
point in space after determining direction. We found
the writing ambiguous (see Cheng & Newcombe, 2005,
for a fuller discussion).
A view of modular subcomponents in the central system
characterizes Cheng's (1986) discussion. Note that we have drawn one memory box, in which both
geometric and featural information are contained. Hence,
this view differs from Wang and Spelke's (2002, 2003) views.
But there is some modularity in the central system, in that
the geometric information is one submodule, the primary one.
Cheng (1986) called it a geometric frame. It encodes the
broad shape of the environment. (In the theory section, we
report evidence indicating that animals may encode far less
than the shape.) On this frame, crucial featural information
may be pasted. Rotational errors ensue when featural
information fails to be entered at all (learning failure),
or fails to be pasted on the geometric frame.
In a completely integrated view (Newcombe, 2002),
featural and geometric information are encoded together in the
central system. Their use may be weighted by many factors,
including reliability of information, stability of
landmarks, etc. Rotational errors ensue when featural
information is either not entered at all into the central
system (learning failure), or if they are weighted very
little (e.g., because they are thought to be unreliable).
One of us (Cheng, 2005) has recently put
forth another view of modularity that takes on elements of all the views in
Figures 91 to 93 (Figure 94). In this view, all spatial information,
geometric and featural, is stored together in one representation, in this
respect resembling Figure 93 and Newcombe's (2002, 2005) views. But at least
one process that operates on the stored information is modular, the
heading-by-axes process. This is a global direction-determining process that
uses global shape parameters derived solely from geometric properties based on
the entire shape of the space. This process is consistent with Wang and Spelke's
(2002) views. Other processes are not modular in this fashion. In particular,
the process of determining an exact location of a target is based on both
featural and geometric information. This process, however, weights
preferentially cues near the target location. Such a view is
foreshadowed in Cheng (1986, p. 172)
A different kind of modularity concerns the use of information
in guiding action, modularity at output. According to
the view shown in Figure 95 (Panel
A), different kinds of information guide action separately.
They do not interact. In contrast, the theory shown in
Figure 95 (Panel B) proposes that different kinds of information guide action together.
The information is integrated at output. We have shown geometric and featural
information in this picture, but the same notion
applies to other kinds of information as well. Here,
we are not concerned with how information is
centrally organized (the ? in A reflects this
uncertainty), but with whether different kinds of
information are integrated at output.
This idea of modularity at output is
best explained with concrete experimental examples.
In the training set up in Figure 96 (left) the search space
is the gray ring. The searcher has the problem of
determining direction: at which direction from the
center of the ring to search at. Around the ring are
distant cues for direction determination. We assume
that the animal is disoriented, but it has
unambiguous geometric cues (the trapezoidal arena)
and a featural cue at the top left corner.
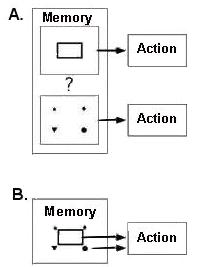 |
Figure 95. Modulartity at Output: A. Modular
Systems. B. Unified System.
|
After sufficient training, the cues
are put in conflict (Figure 96, right panel). The featural cue is moved to
the top right corner. Arrows indicate interesting
theoretical possibilities. The animal might rely
completely on geometric information (left arrow). It
might rely completely on the featural cue (right
arrow). It might vacillate between the locations
dictated by the geometric and the featural cues. Or,
it might average the dictates of geometric and
featural cues, and search somewhere between the
right and left arrows.
This last outcome indicates
integration of geometric and featural information at
output. The vacillation outcome is ambiguous.
It might result from the existence of competing
modules, or it might occur with integrated
representations if the animal computes degree of
match between competing locations and finds two
imperfect matches.
This conceptual paradigm is not limited to geometric and
featural cues. Consider for example an oriented animal
trained in the training set up on the left in Figure 97. Its inertial
sense of direction and the geometric cues of the trapezoidal
arena both give directional cues. The two kinds of cues are put in
conflict on the right, when the trapezoidal space is
rotated in Earth-based coordinates. This puts
geometric and inertial cues in conflict. Thus, many kinds of cues can be
tested in this fashion. One recent theory of the
evolution of spatial cognition (Jacobs & Schenk,
2003) suggests that a part of the hippocampus is
devoted to determining bearing or direction. If we
read these authors right, this bearing system would
use all available directional cues in an integrated
fashion.
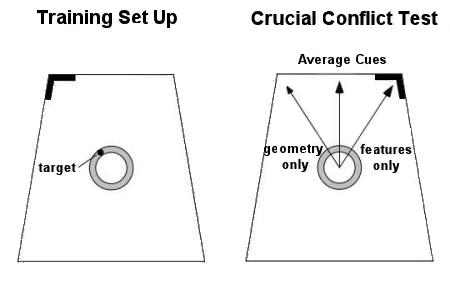 |
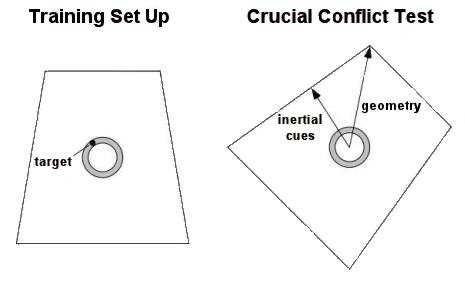 |
Fig 96. Dissociation of Feature and Geometric Cues.
|
Figure 97. Dissociation of Geometric and Inertial Cues.
|
Currently, debate concerning
1) the nature of geometric cues encoded, and 2) the modularity of
geometric encoding form current topics of research. The work
reviewed all use domesticated species or at least animals living
most of their lives in rectilinear indoor spaces. This applies to
the humans, monkeys, rats, pigeons, chicks (although they had few
days of life before being tested), and even the fish in aquaria.
Cheng and Newcombe (2005) wondered what this experience with
rectilinear spaces in development might contribute to the encoding
of geometric and featural cues. Recent research has also spread to
wild-caught birds who have had at least substantial experience
outdoors in natural settings. Some interesting differences have
already been found (Gray et al., 2005), and perhaps more will be
found (e.g., Kelly's program of testing Clark's nutcrackers in
'geometry' paradigms).
Benhamou, S., & Poucet, B. (1998). Landmark use by navigating
rats (Rattus norvegicus): Contrasting geometric and
featural information. Journal of Comparative Psychology,
112, 317-322.
Burgess, N., Jackson, A., Hartley, T., & O'Keefe, J. (2000).
Predictions derived from modeling the hippocampal role in
navigation. Biological Cybernetics, 83, 301-312.
Burgess, N., & O'Keefe, J. (2003). Neural representations in
human spatial memory. Trends in Cognitive Science,
7, 517-519.
Chamizo, V.D. (2003). Acquisition of
knowledge about spatial location: Assessing the generality of
the mechanism of learning. Quarterly Journal of Experimental
Psychology, 56B, 102-113.
Cheng, K. (1986). A purely geometric module
in the rat's spatial representation. Cognition, 23,
149-178.
Cheng, K. (2005a). Reflections on geometry and navigation.
Connection Science,
17, 5-21.
Cheng, K. (2005b). Goldfish matching
geometric and featural cues: A re-interpretation of some of the
data of Vargas et al. (2004). Journal of Comparative
Psychology,
119, 455-457.
Cheng, K., & Gallistel, C.R. (1984). Testing the geometric power
of a spatial representation. In H.L. Roitblat, H.S. Terrace, &
T.G. Bever (Eds.), Animal cognition (pp. 409-423).
Hillsdale, NJ: Erlbaum.
Cheng, K., & Gallistel, C.R. (2005). Shape parameters explain
data from spatial transformations: Comment on Pearce et al.
(2004) and Tommasi, & Polli (2004). Journal of Experimental
Psychology: Animal Behavior Processes, 31, 254-259.
Cheng, K., & Newcombe, N.S. (2005). Is there a geometric module
for spatial orientation? Squaring theory and evidence.
Psychonomic Bulletin & Review, 12, 1-23.
Dudchenko, P. (2003). The head direction
system and navigation. In K.J. Jeffery (Ed.), The
neurobiology of spatial behaviour (pp. 173-186). Oxford:
Oxford University Press.
Egerton, S., Callaghan, V., & Chernett, P.
(2000). A biologically inspired mapping model for autonomous
mobile robots. In M. Mohammadin (Ed.), New frontiers in
computational intelligence and its applications (pp. 20-29).
Amsterdam: IOS Press.
Ekstrom, A.D., Kahana, M.J., Caplan, J.B.,
Fields, T.A., Isham, E.A., Newman, E.L., et al. (2003). Cellular
networks underlying human spatial navigation. Nature,
425, 184-187.
Epstein, R., DeYoe, E.A., Press, D.Z., Rosen,
A.C., & Kanwisher, N. (2001). Neuropsychological evidence for a
topographical learning mechanism in parahippocampal cortex.
Cognitive Neuropsychology, 18, 481-508.
Epstein, R., Graham, K.S., & Downing, P.E.
(2003). Viewpoint-specific scene representations in human
parahippocampal cortex. Neuron, 37, 865-876.
Epstein, R., Harris, A., Stanley, D., &
Kanwisher, N. (1999). The parahippocampal place area:
Recognition, navigation, or encoding? Neuron, 23,
115-125.
Epstein, R., & Kanwisher, N. (1998). A
cortical representation of the local visual environment.
Nature, 392, 598-601.
Fodor, J.A. (1983). The modularity of mind.
Cambridge, MA: MIT Press.
Fritsch, D.S., Pizer, S.M., Morse, B.S.,
Eberly, D.H., & Liu, A. (1994). The multiscale medial axis and
its applications in image registration. Pattern Recognition
Letters, 15, 445-452.
Gallistel, C.R. (1990). The organization
of learning. Cambridge, MA: M.I.T. Press.
Golob, E.J., Stackman, R.W., Wong, A.C., &
Taube, J.S. (2001). On the behavioral significance of head
direction cells: Neural and behavioral dynamics during spatial
memory tasks. Behavioral Neuroscience, 115,
285-304.
Gouteux, S., Thinus-Blanc, C., & Vauclair, J.
(2001). Rhesus monkeys use geometric and non-geometric
information during a reorientation task. Journal of
Experimental Psychology: General, 130, 505-519.
Graham, M., Good, M. A.,
McGregor, A., & Pearce, J. M. (2006). Spatial learning based on
the shape of the environment is influenced by properties of the
objects forming the shape. Journal of Experimental
Psychology: Animal Behavior Processes, 32, 44-59.
Gray, E.R., Bloomfield,
L.L., Ferrey, A., Spetch, M.L., & Sturdy, C.B. (2005). Spatial
encoding in mountain chickadees: Features overshadow geometry.
Biology Letters, 1, 314-317.
Hartley, T., Burgess, N., Lever, C., Cacucci,
F., & O'Keefe, J. (2000). Modelling place fields in terms of the
cortical inputs to the hippocampus. Hippocampus, 10,
369-379.
Hartley, T., Maguire, E.A., Spiers, H.J., &
Burgess, N. (2003). The well-worn route and the path less
travelled: Distinct neural bases of route following and way
finding in humans. Neuron, 37, 877-888.
Hartley, T., Trinkler, I., & Burgess, N.
(2004). Geometric determinants of human spatial memory.
Cognition, 94, 39-75.
Hayward, A., McGregor, A., Good, M.A., &
Pearce, J.M. (2003). Absence of overshadowing and blocking
between landmarks and geometric cues provided by the shape of a
test arena. Quarterly Journal of Experimental Psychology,
56B, 114-126.
Hermer, L., & Spelke, E. (1994). A geometric
process for spatial representation in young children. Nature,
370, 57-59.
Hermer, L., & Spelke, E. (1996). Modularity
and development: The case of spatial reorientation. Cognition,
61, 195-232.
Hermer-Vazquez, L., Spelke, E., & Katsnelson,
A. (1999). Source of flexibility in human cognition: Dual task
studies of space and language. Cognitive Psychology,
39, 3-36.
Huttenlocher, J., & Vasilyeva, M. (2003). How
toddlers represent enclosed spaces. Cognitive Science,
27, 749-766.
Jacobs, L.F., & Schenk, F. (2003). Unpacking
the cognitive map: The parallel map theory of hippocampal
function. Psychological Review, 110, 285-315.
Jeffery, K.J. (Ed.) (2003). The
neurobiology of spatial behaviour. Oxford: Oxford University
Press.
Kelly, D.M., & Spetch, M.L. (2001). Pigeons
encode relative geometry. Journal of Experimental Psychology:
Animal Behavior Processes, 27, 417-422.
Kelly, D.M., & Spetch, M.L. (2004a).
Reorientation in a two-dimensional environment I: Do adults
encode the featural and geometric properties of a
two-dimensional schematic of a room? Journal of Comparative
Psychology, 118, 82-94.
Kelly, D.M., & Spetch, M.L. (2004b).
Reorientation in a two-dimensional environment: II. Do pigeons (Columba
livia) encode the featural and geometric properties of a
two-dimensional schematic of a room? Journal of
Comparative Psychology, 118, 384-395.
Kelly, D.M., Spetch, M.L., & Heth, C.D. (1998).
Pigeon's encoding of geometric and featural properties of a
spatial environment. Journal of Comparative Psychology,
112, 259-269.
Learmonth, A.E., Nadel, L., & Newcombe, N.S.
(2002). Children's use of landmarks: Implications for modularity
theory. Psychological Science, 13, 337-341.
Learmonth, A.E., Newcombe, N.S., &
Huttenlocher, J. (2001). Toddlers' use of metric information and
landmarks to reorient. Journal of Experimental Child
Psychology, 80, 225-244.
Lever, C., Wills, T., Caccucci, F., Burgess,
N., & O'Keefe, J. (2002). Long term plasticity in hippocampal
place-cell representation of environmental geometry. Nature,
416, 90-94.
Leyton, M. (1992). Symmetry, causality,
mind. Cambridge, MA: MIT Press.
Maguire, E.A., Burgess, N., Donnett, J.G.,
Frackowiak, R.S.J., Frith, C.D., & O'Keefe, J. (1998). Knowing
where and getting there: A human navigation network. Science,
280, 921-924.
Maguire, E.A., Burgess, N., & O'Keefe, J.
(1999). Human spatial navigation: Cognitive maps, sexual
dimorphism, and neural substrates. Current Opinion in
Neurobiology, 9, 171-177.
Maguire, E.A., Frith, C.D., Burgess, N.,
Donnett, J.G., & O'Keefe, J. (1998). Knowing where things are:
Parahippocampal involvement in encoding object locations in
virtual large-scale space. Journal of Cognitive Neuroscience,
10, 61-76.
Margules, J., & Gallistel, C.R. (1988).
Heading in the rat: Determination by environmental shape.
Animal Learning & Behavior, 16, 404-410.
Marr, D. (1982). Vision. Cambridge,
MA: MIT Press.
McGregor, A., Jones, P.M.,
Good, M.A., & Pearce, J.M. (2006). Further evidence that rats
rely on local rather than global spatial information to locate a
hidden goal: Reply to Cheng and Gallistel (2005). Journal of
Experimental Psychology: Animal Behavior Processes, 32,
314-321.
Newcombe, N.S. (2002). The
nativist-empiricist controversy in the context of recent
research on spatial and quantitative development.
Psychological Science, 13, 395-401.
Nolfi, S. (2002). Power and limits of
reactive agents. Robotics and Autonomous Systems, 42,
119-145.
O'Keefe, J., & Burgess, N. (1996). Geometric
determinants of the place fields of hippocampal neurons.
Nature, 381, 425-428.
O'Keefe, J., Burgess, N., Donnett, J.G.,
Jeffery, K.J., & Maguire, E.A. (1999). Place cells, navigational
accuracy, and the human hippocampus. In G.W. Humphreys, J.
Duncan, & A. Treisman (Eds.), Attention, space, and action:
Studies in cognitive neuroscience (pp. 153-164). Oxford,
U.K.: Oxford University Press.
O'Keefe, J., & Nadel, L. (1978). The
hippocampus as a cognitive map. Oxford: Oxford University
Press.
Pearce, J.M., Good, M.A., Jones, P.M., &
McGregor, A. (2004). Transfer of spatial behaviour between
different environments: Implications for theories of spatial
learning and for the role of the hippocampus in spatial
learning. Journal of Experimental Psychology: Animal
Behavior Processes, 30(2), 135-147.
Pearce, J.M., Graham, M.,
Good, M.A., Jones, P.M., & McGregor, A. (2006). Potentiation,
overshadowing, and blocking of spatial learning based on the
shape of the environment. Journal of Experimental Psychology:
Animal Behavior Processes, 32, 201-214.
Pearce, J.M., Ward-Robinson, J., Good, M., Fussell, C., & Aydin,
A. (2001). Influence of a beacon on spatial learning based on
the shape of the test environment. Journal of Experimental
Psychology: Animal Behavior Processes, 27, 329-344.
Pizer, S.M., Fritsch, D.S., Yushkevich, P.A.,
Johnson, V.E., & Chaney, E.L. (1999). Segmentation,
registration, and measurement of shape variation via image
object shape. IEEE Transactions on Medical Imaging, 18,
851-865.
Poucet, B., Lenck-Santini, P.P., & Save, E.
(2003). Drawing parallels between the behavioural and neural
properties of navigation. In K.J. Jeffery (Ed.), The
neurobiology of spatial behaviour (pp. 187-198). Oxford:
Oxford University Press.
Sovrano, V.A., Bisazza, A., & Vallortigara, G. (2002).
Modularity and spatial reorientation in a simple mind: Encoding
of geometric and nongeometric properties of a spatial
environment by fish. Cognition, 85, B51-B59.
Sovrano, V.A., Bisazza, A., & Vallortigara, G. (2003).
Modularity as a fish (Xenotoca eiseni) views it:
Conjoining geometric and nongeometric information for spatial
reorientation. Journal of Experimental Psychology: Animal
Behavior Processes, 29, 199-210.
Tommasi, L., Gagliardo, A., Andrew, R.J., &
Vallortigara, G. (2003). Separate processing mechanisms for
encoding geometric and landmark information in the avian brain.
European Journal of Neuroscience, 17, 1695-1702.
Tommasi, L., & Polli, C. (2004).
Representation of two geometric features of the environment in
the domestic chick (Gallus gallus). Animal Cognition,
7, 53-59.
Tommasi, L., & Vallortigara, G. (2000).
Searching for the center: Spatial cognition in the domestic
chick (Gallus gallus). Journal of Experimental
Psychology: Animal Behavior Processes, 26,
477-486.
Tommasi, L., Vallortigara, G., & Zanforlin,
M. (1997). Young chicks learn to localize the center of a
spatial environment. Journal of Comparative Physiology A,
180, 567-572.
Vallortigara, G., Pagni, P., & Sovrano, V.A.
(2004). Separate geometric and non-geometric modules for spatial
reorientation: Evidence from a lopsided animal brain. Journal
of Cognitive Neuroscience, 16, 390-400.
Vallortigara, G., Zanforlin, M., & Pasti, G.
(1990). Geometric modules in animals' spatial representations: A
test with chicks (Gallus gallus domesticus). Journal of
Comparative Psychology, 104, 248-254.
Vargas, J.P., López, J.C., Salas, C., &
Thinus-Blanc, C. (2004). Encoding of geometric and featural
information by goldfish (Carassius auratus). Journal
of Comparative Psychology, 118, 206-216.
Vargas, J.P., Petruso, E.J., & Bingman, V.P.
(2004). Hippocampal formation and geometric navigation in
pigeons. European Journal of Neuroscience, 20, 1937-1944.
Vargas, J.P., & López,
J.C. (2005). Different ways of encoding geometric information by
goldfish (Carassius auratus). Journal of Comparative
Psychology, 119, 458-460.
Wall, P.L., Botly, L.C.P., Black, C.K., &
Shettleworth, S.J. (2004). The geometric module in the rat:
Independence of shape and feature learning in a food finding
task. Learning & Behavior, 32, 289-298.
Wang, R.F., & Spelke, E.S. (2002). Human
spatial representation: Insights from animals. Trends in
Cognitive Sciences, 6, 376-382.
Wang, R.F., & Spelke, E.S. (2003).
Comparative approaches to human navigation. In K.J. Jeffery
(Ed.), The neurobiology of spatial behaviour (pp.
119-143). Oxford: Oxford University Press.
Webb, B. (2000). What does robotics offer
animal behaviour? Animal Behaviour, 60, 545-558.
Yeap, W.K., & Jefferies, M.E. (1999).
Computing representations of the local environment.
Artificial Intelligence, 107, 265-301.
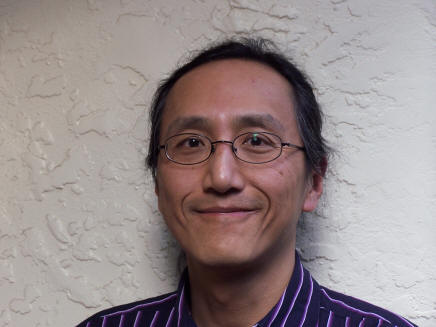 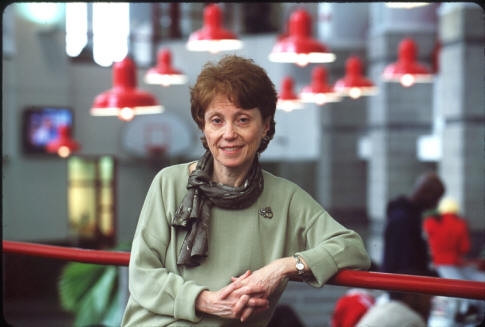
©2006 All
copyrights for the individual chapters are retained by the authors. All other
material in this book is copyrighted by the editor, unless noted otherwise. If
there has been an error with regards to unacknowledged copyrighted material,
please contact the editor immediately so that this can be corrected. Permissions
for using material in this book should be sent to the editor.
|
|
|